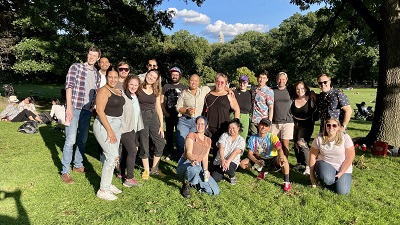
How do we create a methodology to measure team demographics in a way that both provides valuable data, and ensures every team member feels included by the process?I joined Spektrix in 2021 as VP of People, with a background in Talent Development and Learning. In addition to leading global DEI initiatives, I've been a board member for several large employee resource groups and non-profit organizations and have always favored a data-driven approach to leading change.
When I joined, an internal team led by the CEO and co-founder, Michael Nabarro, had made substantial strides in exploring how they could challenge and improve their approach to measuring team demographics. One of my first major undertakings at the company was to work with them to finalize their decisions, and then to distribute and analyze the results of the team demographic survey.
In the summer of 2020 Spektrix published team demographics for the first time in order to take public accountability for our role in improving diversity, equity and inclusion. In doing so, Spektrix was responding to powerful and legitimate demands for better representation and treatment initiated by the Black Lives Matter movement and taken up by voices representing many historically excluded groups. The survey carried out was similar to those run by numerous companies across the globe, and was enough to indicate a need for action to improve team diversity. But since then the company as a whole, and many of the individuals within it, have made substantial strides in their understanding of inclusive practice. That learning identified a dichotomy between the actions needed to effectively measure and report on team demographics, and the actions that would ensure every team member felt equally included and welcome as part of the Spektrix team. At worst, efforts to measure diversity could in themselves be detrimental to the wider effort to improve inclusion.
In this blog, I outline the background of the data presented in An Update on Spektrix Team Demographics: 2021. The language we use to describe demographic characteristics follows decisions taken in the development of the Spektrix Language and Identity guide in 2020. You can find a summary of our approach here, and you’re welcome to reach out to find out more.
Measurement v Inclusion
Why measure diversity at all? It’s the stated goal of Spektrix to build a team that reflects the communities in which our offices are based. Working towards that goal requires three steps:
- Understanding the demographics of our team, in order to
- Identify groups which are underrepresented compared to the communities in which we work, in order to
- Use that data to guide our work to attract, recruit and retain a more diverse team
But in this scenario, there was a risk that in achieving step 1 - the accurate measurement of our team - we undermined step 3 - maintaining and building diversity through the creation of an equitable, inclusive workplace. To illustrate how the two priorities overlap, let’s consider some key factors that have informed company-wide learning over the last year.
- Spektrix has taken guidance from numerous partners and experts around the impact of language on identity and inclusion. Campaigns such as Inc Arts’ #BAMEover showed us that by asking people to assign themselves to categories which were too broad - such as ‘Black, Asian and Minority Ethnic’ (BAME) in the UK, or ‘Black, Indigenous and People of Color’ (BIPOC) in the US, we risk implying similarities of experience which don’t exist, or undermining the individual experience of each respondent.
- The word ‘other’ is itself othering - listing a limited range of categories, and combining everyone else into a generalized pool, again risks undermining individual identity and creating a sense that some identities are of less importance than others.
- Extensive, company-wide work to create a consistent approach to describing Language and Identity concluded that self-description should always be the preferred approach, giving individuals or small groups the right to describe themselves in their own words, rather than choosing from pre-assigned fields.
- In 2020 we measured only four aspects of identity. We’d increased our awareness of the impact of other characteristics including neurodivergence, caring responsibilities and social class on individuals’ lived experience, widening the range of demographics we felt it was important to measure and understand.
From the perspective of inclusion, the ideal scenario might have been to give every individual a blank sheet of paper, and invite them to describe themselves using the language and characteristics they felt were most important to their own identity. But we also needed data which would allow us to measure movement over time, compare our team demographics to those of the communities in which we work, and identify individual and intersectional areas for improvement. All of these considerations made it essential that we found a way to combine individual identities into aggregated groups, without assuming, marginalizing or otherwise undermining the individuality of any team member.
Our Approach
After internal discussion and external advice, we settled on an approach that felt like a good starting place for this first attempt at inclusive measurement. We’re deliberately not describing this as a solution. We’re reasonably confident that our approach is more inclusive than it was in 2020; we’re working to do better still in 2022, and no doubt there’ll be many more lessons we can learn in the longer term. We began by agreeing on a set of key principles which balanced the importance of self-description with the need for measurable data, and which helped the whole team understand our choices.
We agreed, first, to be transparent about the problem we’d identified, the choices we’d made, and the reasons for those decisions. We benefited from the fact that Spektrix is a data-led company, and that many of our team members talk daily about the importance of segmentation and aggregated information to drive continual improvement; nevertheless, we worked hard to ensure we explained our approach in plain language, without excuses or apologies, and that we invited every team member to ask questions if they wished. These explanations prefaced the survey, and introduced each individual question. We’ve included brief versions of some of these explanations in the parallel ‘Team Demographics’ blog.
We then identified three question types or approaches.
Single-answer questions
For three questions relating to social class, age and transgender status, we invited people to choose a single checkbox from pre-defined criteria. In the first instance, we were trialing the use of a structure developed by the UK’s Social Mobility Commission. We used the same framework to aggregate seven options into the three socio-economic groups shared on this blog. So far, this approach is working well to help us measure and report on a complex concept, and team members appear to have understood and engaged with the question positively.
The age question offered a range of clear, numeric answers. In asking “Are you transgender?”, we separated transgender status from a broader question about gender and offered a simple ‘Yes/No’ answer. In all three cases, people were given the option of ‘prefer not to say’.
Checkboxes with free text option
The majority of questions - those on nationality, languages, sexual orientation, caring responsibilities, disability and neurodivergence - were presented as a set of pre-defined categories, with the option for people to self-define using a free text field. This approach was chosen for one of two reasons:
- Because there was general, global consensus on the categories which should be offered in each area, and these felt relatively distinct and well-defined. We used Yes/No questions to ask if people considered themselves disabled or neurodivergent, or if they had caring responsibilities. When asking about sexual orientation, we identified four options, based on guidance from Stonewall, and found that only 1.7% of team members told us they didn't feel they belonged to any of these groups. We’ll use the self-defined responses to add or amend categories in the future if necessary.
- Because there were a potentially infinite number of responses, and it felt redundant to list them all out. When asking about nationality(ies) and language(s) spoken at home, we offered checkboxes for American or British, and for English, based on the location of our offices. We’ve shared these data as free text responses, as we’re interested in the range of identities across our organization, what that tells us about our team, and how it might influence the support we offer or the global events we mark internally.
Self-definition followed by self-aggregation
There were two questions - race/ethnicity and gender - where we were less confident that we could capture individuals’ unique identities using a set of pre-defined criteria, and where we were particularly aware of the potential to marginalize or otherwise cause harm to members of the team by excluding their identity from the options offered. For these questions we reversed the approach described above, breaking each question down into two parts.
In the first, we promoted self definition by offering a free text field - demonstrating to every respondent that their individual identity is important, and giving us rich contextual data to refine the categories offered in the future.
In the second part, we asked individuals to choose from a set of pre-defined categories themselves - always with the option to tell us if none of those choices felt appropriate. By putting this choice into their hands we avoided falsely aggregating discrete identities, whilst ensuring that the majority of individuals were assigned to measurable groups and quickly identifying categories that should be included in future surveys.
For the question on gender, free text responses aligned closely with the categories offered, and 99.1% of team members told us they belonged to one of the pre-defined groups. The responses to the question on race and ethnicity were more complex, and are outlined in more detail below.
Race and Ethnicity
The question, “Which of the following categories best describes your race and/or ethnicity?” was the only one in which a substantial number of people chose to self-identify in ways which did not map well to the choices we had offered. Others told us that the categories we offered may have missed nuance in reflecting every individual’s identity or in identifying people from groups which have experienced exclusion and/ or racism.
The ten pre-defined categories had been developed from US and UK census groups, further informed by our own work around inclusive language and the data collected from our team in 2020.
115 people gave a total of 38 distinct free-text responses, which are listed alongside the aggregated results for this question. These responses showed us that race, nationality and ethnicity intersect to create individual identities in ways that defy many methods of categorization. It’s important to us to track how well our team represents the diversity of the communities in which we work, but it’s more important to ensure we avoid perpetuating harm to people who have, or who belong to communities which have, experienced racism and/or exclusion.
We’ll be taking active steps in future years to better reflect the intersectionality of race, nationality and ethnicity; to build our understanding of our team as a whole; to positively include every individual within that team; and to effectively measure our progress against our diversity goal.
Specifically:
- We have retrospectively introduced a new category of ‘Self identified’ within the options relating to the question about race and/or ethnicity. This will help us to understand the number of people who felt the categories offered did not reflect their individual identity.
- We’ll review how we ask about race, ethnicity and nationality, and trial new approaches with the aim of better reflecting every team member’s experience and of better understanding the makeup of our team.
- We’ll add ‘Jewish’ as a category within any question/s about race and/or ethnicity. In practice, 100% of people in the ‘self identified’ category this year identified as Jewish when invited to self-describe.
- We’ll identify communities within the broader category whose members may have experienced racism and/or exclusion, and consider whether they should be separately recognized within the data.
Presentation of Data
When collecting and sharing highly personal data, every choice can carry connotations. We were initially drawn to pie charts, which we felt gave a positive sense of every individual belonging to a collective whole; but we came to realize that 100% bar charts made it easier to contrast data between questions, give visibility to small data sets, and to compare results year-on-year in the future. Similarly, color and sequence can convey both positive and negative meaning; we have listed every result in alphabetical or logical order (e.g. age is listed from youngest to oldest), and assigned our brand colors at random to each response.
Learnings and Next Steps
This year’s experience has provided a strong starting point for us to continue improving the way we measure individuals’ personal characteristics - whether we’re measuring the demographics of our own team, of applicants for the jobs we advertise, or working with cultural organizations to measure the diversity of their audiences.
We’ve identified immediate areas for improvement in 2022, and it’s likely there’ll be further changes as we continue to analyze this year’s data. Those learnings will impact our approach to measurement, but more importantly they’ll inform our recruitment, management and learning to support the long-term diversity of our team. The next step in this process is to spend time exploring the raw data from the survey, identifying intersectionality between the responses to different questions, and mapping that data to recruitment, length of service, workplace satisfaction and more. Over time, this will indicate the success of our approach to diversity, equity and inclusion, identify key areas for improvement, and provide a baseline for future growth.
If you’re interested in engaging with our work around demographic measurement and growth, please continue to explore our site or reach out for a conversation.
Explore our Values and how we’re putting them into practice
Spektrix Values
Zack Moore is VP of People at Spektrix